Improving Cardinality Analysis

By
Ashish Hanwadikar
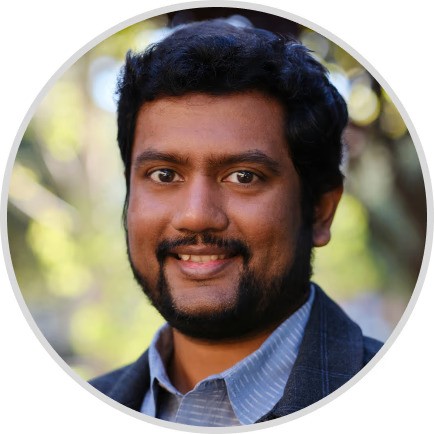
By
Pralav Dessai
Published on
Aug 27, 2024
Table of Contents
In our earlier blogs, we explored how high cardinality data enhances observability by providing detailed insights into system performance and user behavior. While the granularity of this data enables in-depth analysis and segmentation of operational metrics, managing high cardinality data can introduce its own set of challenges and costs.
In this blog, we'll outline strategies to manage these costs effectively while still reaping the benefits of high cardinality data. Effective cardinality optimization involves reducing the number of unique values in a dataset to alleviate resource constraints, improve query performance, and simplify data processing. Here’s how to approach it:
Cardinality Analysis:
By tracking cardinality sources and monitoring churn, resources can be strategically allocated to critical data points or timelines. For example, observing fluctuations in unique user sessions accessing a web application can reveal potential performance issues with a particular service or shifts in user behavior during seasonal changes. This enables efficient allocation of data collection and compute resources for targeted analysis across specific timeframes or user segments.
Sorting:
Sorting attributes by their cardinality can provide insights into system behavior. For example, in a monitoring setup for a cloud-based application, sorting error codes by frequency can highlight which errors are most prevalent, to help prioritize efforts towards resolving the most critical issues impacting system performance.
Removing Unnecessary Attributes:
Eliminating attributes that aren't essential for diagnosis helps to streamline observability and root cause analysis. For instance, in a log monitoring system, excluding verbose debugging details rarely used for troubleshooting simplifies log data and facilitates faster identification of critical events.
Filtering High Cardinality Attributes:
Another effective strategy involves minimizing the volume of data within high cardinality attributes. For instance, rather than storing separate log entries for each request, only recording log entries based on factors such as error type can significantly reduce data volume. This approach not only conserves storage space but also accelerates the analysis of server performance and troubleshooting efforts.
Data Transformation:
Data shaping techniques will reduce high cardinality into more manageable forms without sacrificing critical information.
Data Mapping:
This approach reduces high cardinality attributes to lower cardinality equivalents. For instance, converting IP addresses to geographic regions or grouping service names into broader categories enables pattern detection across various regions or service types without keeping track of less meaningful IP addresses.
Data Aggregation:
Aggregating data is particularly effective for managing high cardinality metrics. By applying recording rules during data ingestion (e.g., sum, count, min, max), metrics can be pre-aggregated, significantly reducing the number of distinct data points stored. This optimization not only enhances query performance but also streamlines data handling and analysis processes.
Generating Metrics from Events:
Transforming unstructured event data into structured metrics can reduce its cardinality and optimize analysis during troubleshooting. For example, if event data includes service names, timestamps, response times, and contextual details like client IP and user agent, calculating metrics such as average response times for each service can provide actionable insights while also reducing cardinality.
By implementing these methods, organizations can manage high cardinality effectively, optimize resource utilization, improve query performance, and streamline data processing and analysis workflows.
In our next blog we will talk about the storage considerations for high cardinality data.
Stay tuned!